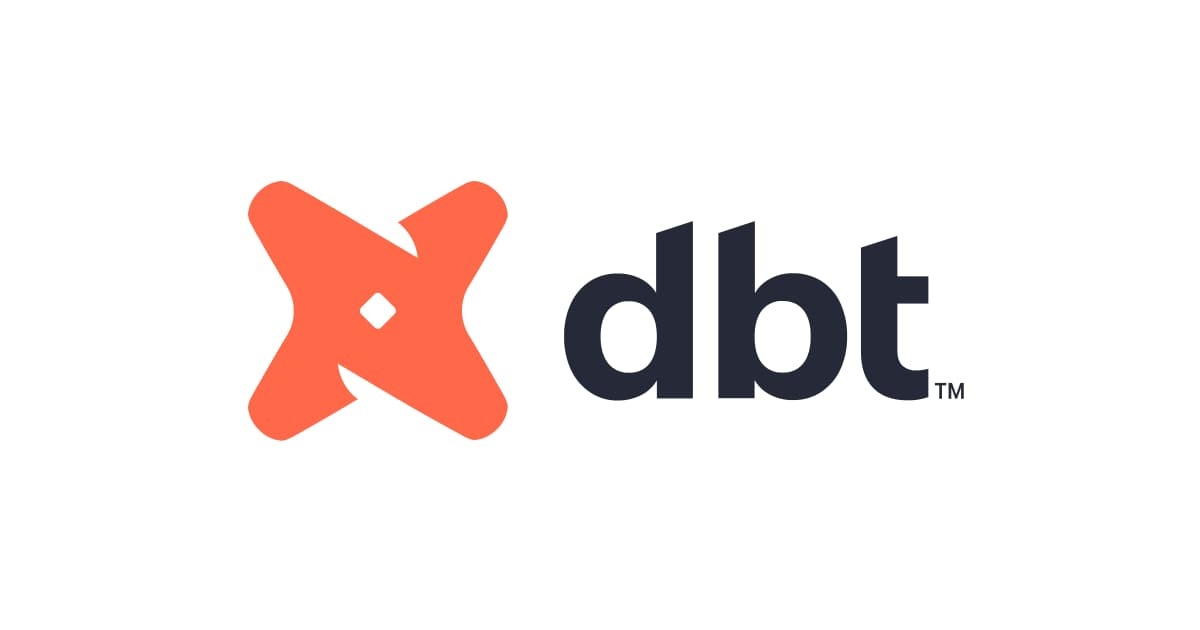
dbt-athenaのHiveテーブル向けのIncremental modelsについて、どのようにデータとGlueテーブルが作成されるのか調べてみた
データアナリティクス事業本部 機械学習チームの鈴木です。
機械学習モデル開発用のデータマート作成に、dbt-athenaを使いたく、どんな感じで使えそうか試行錯誤中です。
dbtでは積み上げのデータをIncremental modelsで実装することができます。
dbt-athenaでもこの機能はサポートされています。
dbt-athenaは記事執筆時点でHiveとIcebergのテーブルをサポートしていますが、今回はHiveテーブルでのIncremental modelsの機能について、その動作やinsert_overwrite
とappend
の2つのstrategyの違いについて調べたのでまとめました。
dbt-athenaのIncremental models
dbt-athena-communityのGitHubレポジトリの、Incremental modelsのセクションを見ると、3つのstrategyがあることが分かります。
- insert_overwrite
- append
- merge
GitHubレポジトリにも記載がありますが、Hiveテーブルでサポートしているstrategyはinsert_overwrite
とappend
になります。デフォルトはinsert_overwrite
ですが、partitioned_by
がconfigで設定されていない場合はappend
と同じになります。
環境構築
dbt実行環境
以前公開した以下の記事の方法でEC2上にdbt-coreとdbt-athena-communityをインストールして検証しました。
dbtのバージョンは以下になります。
- dbt Core:1.5.6
- dbt-athena-community:1.5.1
Glueテーブル
ソーステーブルとするGlueテーブルを作成しておきました。
今回はincremental
のマテリアライゼーションのモデルを、ソーステーブルのデータを使って作成するケースを考えます。最初にソーステーブルにデータを入れておき、1度モデルを実行した後、データをソーステーブルに追加して、2度目の実行がどうなるか確認しました。
まずテーブル定義は以下のようにしました。
-- 検証用のS3バケット名は適宜置き換えてください。 CREATE EXTERNAL TABLE purchase_records ( id int, customer_id int, purchase_date string, item_name string, quantity int, unit_price int ) ROW FORMAT SERDE 'org.apache.hadoop.hive.ql.io.parquet.serde.ParquetHiveSerDe' STORED AS INPUTFORMAT 'org.apache.hadoop.hive.ql.io.parquet.MapredParquetInputFormat' OUTPUTFORMAT 'org.apache.hadoop.hive.ql.io.parquet.MapredParquetOutputFormat' LOCATION 's3://検証用のS3バケット名/dbt-athena-incremental-raw'
最初から入れておくデータは以下のように準備しました。
insert into purchase_records(id, customer_id, purchase_date, item_name, quantity, unit_price) values (1, 101, '2023-1-1', 'りんご', 4, 200), (2, 102, '2023-1-2', 'バナナ', 3, 150), (3, 103, '2023-1-3', 'みかん', 5, 50), (4, 104, '2023-1-4', 'パイナップル', 1, 300), (5, 105, '2023-1-5', 'メロン', 1, 1000), (6, 106, '2023-1-6', 'ぶどう', 3, 500), (7, 107, '2023-1-7', 'いちご', 1, 200), (8, 108, '2023-1-8', 'キウイ', 4, 100), (9, 109, '2023-1-9', 'レモン', 4, 150), (10, 110, '2023-1-10', 'オレンジ', 3, 200);
追加のデータは以下のように準備しました。
insert into purchase_records(id, customer_id, purchase_date, item_name, quantity, unit_price) values (11, 111, '2023-1-10', 'マンゴー', 3, 700), (12, 112, '2023-1-11', 'アボカド', 2, 300), (13, 113, '2023-1-12', '桃', 3, 200), (14, 114, '2023-1-13', 'サクランボ', 1, 400), (15, 115, '2023-1-14', 'ブルーベリー', 5, 150), (16, 116, '2023-1-15', 'ラズベリー', 2, 180), (17, 117, '2023-1-16', 'ゴールドキウイ', 2, 200), (18, 118, '2023-1-17', 'パッションフルーツ', 2, 250), (19, 119, '2023-1-18', 'パパイヤ', 1, 300), (20, 120, '2023-1-19', 'グレープフルーツ', 1, 220);
追加のデータのポイントとして、ハイライト箇所があります。このレコードはpurchase_date
が最初からテーブルに入っているデータと重複しています。
insert_overwrite
のstrategyでは、append
と異なり、同一パーティションのデータが追加で作成された場合にパーティション内のデータを上書きします。このレコードを使って、strategyを変えた場合に、最終的にできるモデルのレコード数が変わるかを確認することで、strategyの違いについて調べます。
ソースおよびモデルの定義
続いて、検証のために準備したソースおよびモデルの定義について確認します。
今回は、先ほど記載したGlueテーブルに対応するソーステーブル1つを参照する、1つのモデルを実行し、Glueデータベース上に作成されるテーブルの中身がどのように変わるか検証しました。
ただし、モデルのIncremental modelsの設定は以下の3パターンを試したため、モデルの定義は3つ分記載します。
- パーティション分割なしの場合
- パーティション分割ありでstrategyが
insert_overwrite
の場合 - パーティション分割ありでstrategyが
append
の場合
ソーステーブル
version: 2 sources: - name: raw_tables database: awsdatacatalog schema: cm-nayuts-sample-db tables: - name: purchase_records
モデル(パーティション分割なし)
materialized='incremental'
だけ指定しておきました。ブログ冒頭のガイドを参考に、is_incremental()
を使ってIncremental modelsを表現しました。
{{ config( materialized='incremental' ) }} select id, customer_id, item_name, quantity, unit_price, quantity*unit_price as total_price, purchase_date from {{ source('raw_tables','purchase_records') }} {% if is_incremental() %} where id > (select max(id) from {{ this }}) {% endif %}
モデル(パーティション分割あり、insert_overwrite)
config()
でIncremental modelsであることに加え、strategyがinsert_overwrite
で、purchase_date
の値をキーにパーティション分割することを指定しました。purchase_date
カラムはパーティション分割に使うので、モデルで実行するSELECT文では最後にしました。
{{ config( materialized='incremental', incremental_strategy='insert_overwrite', partitioned_by=['purchase_date'] ) }} select id, customer_id, item_name, quantity, unit_price, quantity*unit_price as total_price, purchase_date from {{ source('raw_tables','purchase_records') }} {% if is_incremental() %} where id > (select max(id) from {{ this }}) {% endif %}
モデル(パーティション分割あり、append)
config()
でstrategyはappend
としました。
{{ config( materialized='incremental', incremental_strategy='append', partitioned_by=['purchase_date'] ) }} select id, customer_id, item_name, quantity, unit_price, quantity*unit_price as total_price, purchase_date from {{ source('raw_tables','purchase_records') }} {% if is_incremental() %} where id > (select max(id) from {{ this }}) {% endif %}
やってみる
パーティション分割なしの場合
dbt run
で初回実行した際にAthenaで実行されたSQLは以下の2つでした。
-- /* {"app": "dbt", "dbt_version": "1.5.6", "profile_name": "test_project", "target_name": "dev", "node_id": "model.test_project.purchase_records_with_total_price"} */ create table "awsdatacatalog"."cm-nayuts-sample-db"."purchase_records_with_total_price" with ( table_type='hive', is_external=true,external_location='s3://s3_data_dirで指定したパス/cm-nayuts-sample-db/purchase_records_with_total_price/98c5d8a5-4bfe-422c-bb99-eca916620b75', format='parquet' ) as select id, customer_id, item_name, quantity, unit_price, quantity*unit_price as total_price, purchase_date from "awsdatacatalog"."cm-nayuts-sample-db"."purchase_records"
※ s3_data_dir
で指定したパスは~/.dbt/profiles.yml
で指定したs3_data_dir
キーのバリューのことです。
alter table `cm-nayuts-sample-db`.`purchase_records_with_total_price` set tblproperties ('classification' = 'parquet')
続いて、purchase_records
テーブルに追加のデータを入れた後にdbt run
を実行した際のSQLです。
-- /* {"app": "dbt", "dbt_version": "1.5.6", "profile_name": "test_project", "target_name": "dev", "node_id": "model.test_project.purchase_records_with_total_price"} */ create table "awsdatacatalog"."cm-nayuts-sample-db"."purchase_records_with_total_price__dbt_tmp" with ( table_type='hive', is_external=true,external_location='s3://s3_data_dirで指定したパス/cm-nayuts-sample-db/purchase_records_with_total_price__dbt_tmp/e14422e0-e03d-4fbd-b21a-f1d31b1ccade', format='parquet' ) as select id, customer_id, purchase_date, item_name, quantity, unit_price, quantity*unit_price as total_price from "awsdatacatalog"."cm-nayuts-sample-db"."purchase_records" where id > (select max(id) from "awsdatacatalog"."cm-nayuts-sample-db"."purchase_records_with_total_price")
-- /* {"app": "dbt", "dbt_version": "1.5.6", "profile_name": "test_project", "target_name": "dev", "node_id": "model.test_project.purchase_records_with_total_price"} */ insert into "awsdatacatalog"."cm-nayuts-sample-db"."purchase_records_with_total_price" ("id", "customer_id", "purchase_date", "item_name", "quantity", "unit_price", "total_price") ( select "id", "customer_id", "purchase_date", "item_name", "quantity", "unit_price", "total_price" from "awsdatacatalog"."cm-nayuts-sample-db"."purchase_records_with_total_price__dbt_tmp" )
purchase_records_with_total_price__dbt_tmp
テーブルに増分を一度格納し、モデルにINSERTしていることが分かりました。
なお、purchase_records_with_total_price__dbt_tmp
テーブルが作られていますが、CloudTrailからログを確認すると、APIよりdbt-athenaによって削除されるようでした。
ちなみに、できたモデルのデータ件数は20件でした。パーティション分割していない場合はデフォルトのinsert_overwrite
でも挙動はappend
と同じでただの追加になるため、10件 + 10件で合計20件なのは想定通りです。
パーティション分割あり、insert_overwriteの場合
続いて、purchase_date
カラムをpartitioned_by
で指定し、insert_overwrite
で指定した場合です。
初回のモデル実行の際に実行されたSQLは以下でした。
-- /* {"app": "dbt", "dbt_version": "1.5.6", "profile_name": "test_project", "target_name": "dev", "node_id": "model.test_project.purchase_records_with_total_price"} */ create table "awsdatacatalog"."cm-nayuts-sample-db"."purchase_records_with_total_price" with ( table_type='hive', is_external=true,external_location='s3://s3_data_dirで指定したパス/cm-nayuts-sample-db/purchase_records_with_total_price/2031a80f-4fcf-4396-aedf-40293db5716e', partitioned_by=ARRAY['purchase_date'], format='parquet' ) as select id, customer_id, item_name, quantity, unit_price, quantity*unit_price as total_price, purchase_date from "awsdatacatalog"."cm-nayuts-sample-db"."purchase_records"
alter table `cm-nayuts-sample-db`.`purchase_records_with_total_price` set tblproperties ('classification' = 'parquet')
続いて、purchase_records
テーブルに追加のデータを入れた後、モデル実行の際に実行されたSQLです。
-- /* {"app": "dbt", "dbt_version": "1.5.6", "profile_name": "test_project", "target_name": "dev", "node_id": "model.test_project.purchase_records_with_total_price"} */ create table "awsdatacatalog"."cm-nayuts-sample-db"."purchase_records_with_total_price__dbt_tmp" with ( table_type='hive', is_external=true,external_location='s3://s3_data_dirで指定したパス/cm-nayuts-sample-db/purchase_records_with_total_price__dbt_tmp/82893541-0f86-4e20-9c16-241215d4845d', partitioned_by=ARRAY['purchase_date'], format='parquet' ) as select id, customer_id, item_name, quantity, unit_price, quantity*unit_price as total_price, purchase_date from "awsdatacatalog"."cm-nayuts-sample-db"."purchase_records" where id > (select max(id) from "awsdatacatalog"."cm-nayuts-sample-db"."purchase_records_with_total_price")
-- /* {"app": "dbt", "dbt_version": "1.5.6", "profile_name": "test_project", "target_name": "dev", "node_id": "model.test_project.purchase_records_with_total_price"} */ select distinct purchase_date from "awsdatacatalog"."cm-nayuts-sample-db"."purchase_records_with_total_price__dbt_tmp"
-- /* {"app": "dbt", "dbt_version": "1.5.6", "profile_name": "test_project", "target_name": "dev", "node_id": "model.test_project.purchase_records_with_total_price"} */ insert into "awsdatacatalog"."cm-nayuts-sample-db"."purchase_records_with_total_price" ("id", "customer_id", "item_name", "quantity", "unit_price", "total_price", "purchase_date") ( select "id", "customer_id", "item_name", "quantity", "unit_price", "total_price", "purchase_date" from "awsdatacatalog"."cm-nayuts-sample-db"."purchase_records_with_total_price__dbt_tmp" )
こちらも、一時的なテーブルを作成して増分を格納し、そこからさらにモデルのテーブルにデータをINSERTしていることが分かりました。
ただしここはSQLだけ見ても全容が掴めなかったので、GitHubレポジトリのソースコードとCloudTrailログも見ていきました。
まず、重複したパーティションのデータは、boto3を使って重複したパーティションのデータは削除していることが伺えました。incremental.sql
ファイルのmacroの定義から、strategyがinsert_overwrite
でかつパーティション分割がされている場合には、delete_overlapping_partitions
というヘルパー関数を呼び出していることが分かるためです。
パーティションメタデータはCloudTrailログよりBatchCreatePartitionアクションを実行していることが確認できました。
モデルの件数はデータ件数は19件でした。
2023-1-10
のパーティションを見ると、たしかに上書きされていますね。元々2023-1-10
のパーティションには1件のデータがあったため、20件から1件上書き時に削除されて19件なので想定通りです。
パーティション分割あり、appendの場合
最後にパーティション分割あり、append
の場合です。
初回のモデル実行の際に実行されたSQLは以下でした。
-- /* {"app": "dbt", "dbt_version": "1.5.6", "profile_name": "test_project", "target_name": "dev", "node_id": "model.test_project.purchase_records_with_total_price"} */ create table "awsdatacatalog"."cm-nayuts-sample-db"."purchase_records_with_total_price" with ( table_type='hive', is_external=true,external_location='s3://s3_data_dirで指定したパス/cm-nayuts-sample-db/purchase_records_with_total_price/9d05fc3e-ff0b-48df-b917-8ec65714bddf', partitioned_by=ARRAY['purchase_date'], format='parquet' ) as select id, customer_id, item_name, quantity, unit_price, quantity*unit_price as total_price, purchase_date from "awsdatacatalog"."cm-nayuts-sample-db"."purchase_records"
alter table `cm-nayuts-sample-db`.`purchase_records_with_total_price` set tblproperties ('classification' = 'parquet')
続いて、purchase_records
テーブルに追加のデータを入れた後、モデル実行の際に実行されたSQLです。
-- /* {"app": "dbt", "dbt_version": "1.5.6", "profile_name": "test_project", "target_name": "dev", "node_id": "model.test_project.purchase_records_with_total_price"} */ create table "awsdatacatalog"."cm-nayuts-sample-db"."purchase_records_with_total_price__dbt_tmp" with ( table_type='hive', is_external=true,external_location='s3://s3_data_dirで指定したパス/cm-nayuts-sample-db/purchase_records_with_total_price__dbt_tmp/c14d11dc-9d06-422c-864d-5a8d8cee0608', partitioned_by=ARRAY['purchase_date'], format='parquet' ) as select id, customer_id, item_name, quantity, unit_price, quantity*unit_price as total_price, purchase_date from "awsdatacatalog"."cm-nayuts-sample-db"."purchase_records" where id > (select max(id) from "awsdatacatalog"."cm-nayuts-sample-db"."purchase_records_with_total_price")
-- /* {"app": "dbt", "dbt_version": "1.5.6", "profile_name": "test_project", "target_name": "dev", "node_id": "model.test_project.purchase_records_with_total_price"} */ insert into "awsdatacatalog"."cm-nayuts-sample-db"."purchase_records_with_total_price" ("id", "customer_id", "item_name", "quantity", "unit_price", "total_price", "purchase_date") ( select "id", "customer_id", "item_name", "quantity", "unit_price", "total_price", "purchase_date" from "awsdatacatalog"."cm-nayuts-sample-db"."purchase_records_with_total_price__dbt_tmp" )
件数は20件でした。
2023-1-10
のパーティションを見ると、追加されて2件となっていました。削除された分がないので、合計20件は想定通りですね。
最後に
dbt-athena-communityでHiveテーブルのIncremental modelsを作った際に、設定によってデータのでき方がどのように変わるか、テーブルの中身や実行されるSQL・リクエストから確認してみました。
モデルでパーティション分割の設定をしている場合は、config()
のstrategyの設定によってパーティションのデータが上書きされる可能性があるのでよく考えて設定する必要があることが分かりました。
また、パーティションメタデータの追加や上書きの処理はSQLだけではなくAWS SDKも組み合わせて使っていることを確認でき、dbt-athena-communityの内部実装を確認する良い機会にもなりました。
今回はHiveテーブルでしたが、dbt-athenaはIcebergテーブルにも対応しているので、そちらについても確認してみたいと思います。